Finding Mislabels in Healthcare AI with Hirundo: VinDr-CXR Case Study
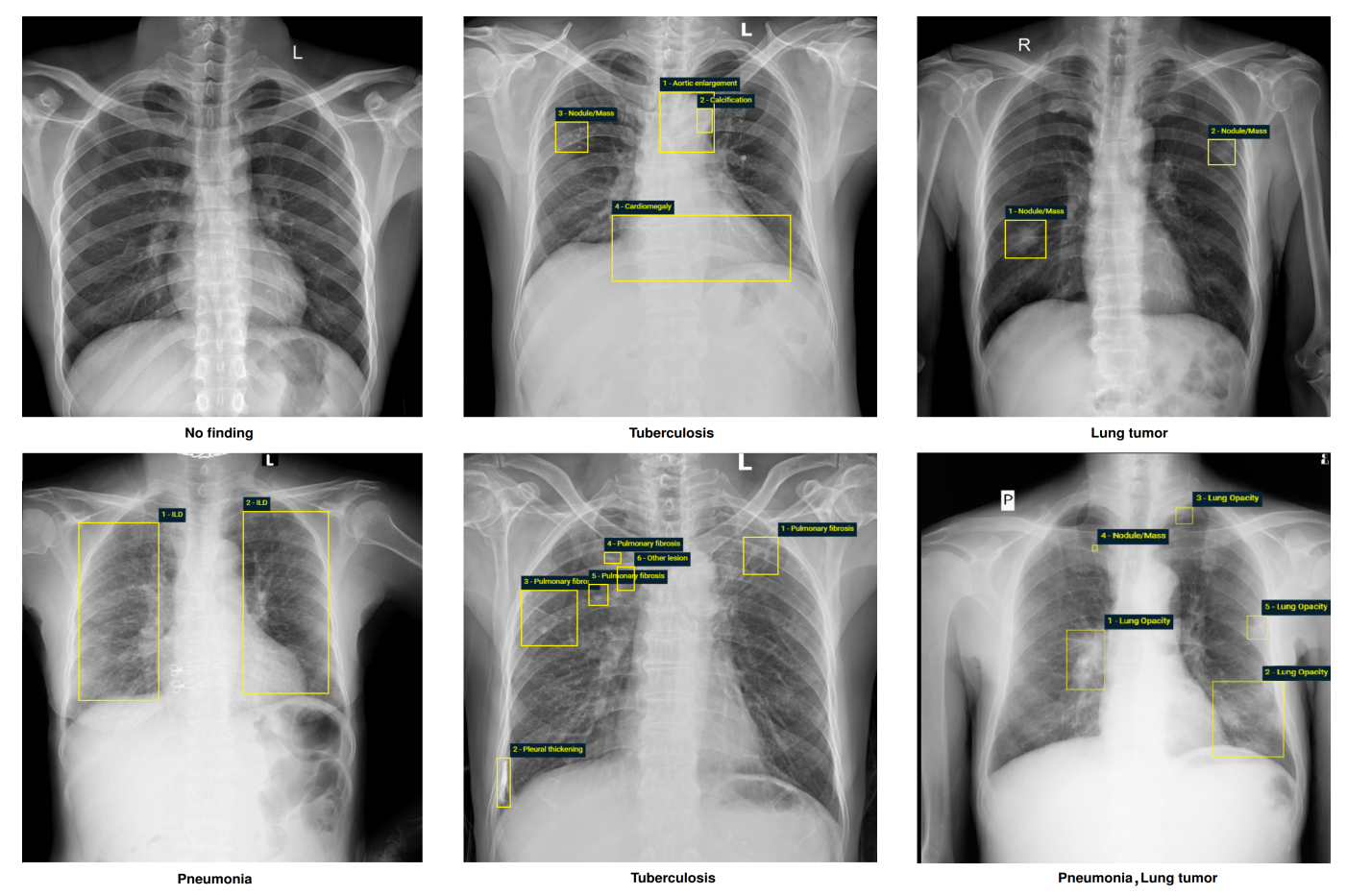
TL;DR
VinDr-CXR is one of the largest and most trusted public datasets of chest x-ray scans (CXR). It features over 18,000 CXRs, annotated by 17 radiologists with at least 8 years of professional experience. Using Hirundo's data influence engine, we were able to automatically locate mislabels/misdiagnoses in 4.2% of the dataset - as verified by certified radiologists.
What is VinDr-CXR and Why it Matters
VinDr-CXR is a comprehensive public dataset, covering a broad range of chest X-ray images annotated by radiologists for the purpose identifying and classifying common lung diseases. Created by the Vingroup Big Data Institute (VinBigData), this dataset comprises more than 18,000 CXR scans from two major hospitals in Vietnam, collected between 2018 and 2020. The images have been detailed for the presence of 28 different radiographic findings and diagnoses. Each scan in the training set was annotated by a group of three radiologists, while the test set saw involvement from five experienced radiologists, whose consensus served as the gold standard for the test labels.
The VinDr-CXR dataset is critically important for the medical field, as one of the most detailed and well-covered public datasets, open for the medical community and the general public. It offers a robust tool for advancing AI technology in medical diagnostics, which in turn improves patient outcomes through more accurate and efficient disease diagnosis.
How Data Quality and Mislabels Risk Patient Outcomes
The quality of data in medical datasets like VinDr-CXR directly influences the performance of AI diagnostic tools. High-quality, accurately labeled data ensures that AI models are trained on reliable information, leading to precise diagnostic outcomes. Conversely, the presence of mislabeled data can significantly degrade the effectiveness of these models. Mislabels can lead to incorrect diagnoses, potentially delaying appropriate medical intervention and affecting patient outcomes adversely.
It is crucial, therefore, for datasets to undergo rigorous quality checks and validation by experienced professionals and data quality tools to minimize errors and maximize the potential benefits of AI in healthcare. In the case of VinDr-CXR, this effort was seen in their use of many expert radiologists, and consensus labeling to reduce the risk of misdiagnosis by individual annotators.
A sample of ten CXR scans analyzed by the Hirundo platform
Finding Mislabels in VinDr-CXR with Hirundo
At Hirundo, a large part of our work is dedicated towards improving AI datasets in critical fields - and it doesn't get more critical than medical diagnosis. Despite VinBigData's rigorous quality assurance and consensus labeling processes, we were still able to find a significant amount of mislabels in this dataset. That is the nature of working with big data - annotation tasks are difficult at scale, and finding mislabels is like finding a needle in a haystack.
Our platform is using a proprietary data influence engine, that traces the impact of individual data samples across the training lifecycle of machine learning models. This innovative technique allows us to pinpoint inaccuracies with unprecedented precision, avoiding the faults of any other known method to refine datasets (like statistical analysis, consensus labeling, using external pre-trained models, and more).
We analyzed 4394 CXR scans - all of the samples in the VinDr-CXR's training set that included findings. Our platform automatically found 5.2% of these samples to be highly suspicious of including mislabels.
The platform didn't just flag which scans are likely to include mislabels - it also pinpointed which bounding boxes (i.e. identified "objects" in each scan) are the suspicious ones. In essence, it means that our analysis not only works on finding issues in datasets for classification models, but also (among others) on object detection models.
We are experts in optimizing AI models and datasets - but we are not experts in the medical field. To verify our findings, we sent a sample of the flagged x-ray scans (along some non-suspicious images, as control group) to an expert radiologist with over 30 years of experience, for an impartial analysis. They found that 80% of the images flagged by our platform to indeed include misdiagnoses. The remaining 20%, while could be of correct diagnosis, were flagged by our platform because they are perceived by the AI model as outliers, and could also lead to adverse effects on its accuracy.
Note: for further access to our findings, please reach out to us at info@hirundo.io
Conclusion
The VinDr-CXR dataset exemplifies the critical importance of high-quality, well-annotated data in the realm of medical AI research. As one of the largest publicly accessible chest X-ray datasets, it not only supports the development of advanced diagnostic tools, but also underscores the challenges inherent in managing large-scale medical data.
Using Hirundo's unique data influence engine, we were able to automatically find a significant amount of mislabels in a sample this trusted dataset. 4.2% of the CXR scans analyzed had mislabeled diagnoses, as verified by external radiology experts who reviewed our findings.
Our effort to identify and correct mislabels highlights the dynamic nature of AI in healthcare - where continuous improvement is key to ensuring that AI can reliably assist in patient diagnosis and treatment. This collaborative approach between AI technology and medical expertise promises to enhance the accuracy and effectiveness of medical diagnostics, ultimately leading to better patient care and outcomes. We believe that by combining the expertise of the medical community, along with our state of the art technology, we could bring AI in healthcare to its next stage.
About Hirundo
We are a TLV/London based startup, offering an AI Optimization & Machine Unlearning platform, allowing AI teams to quickly find and remove any unwanted and faulty data powering their AI/ML models.
The Hirundo platform uses state-of-the-art technology, combining proprietary methods and best-in-class open source solutions. Our team includes experienced professionals from academia and industry, including the Technion’s former Dean of Computer Science.
We have partnered with some of industry’s leading companies - including Intel, Nvidia, Microsoft, Google and Amazon - and are starting to work with further early adopters looking to revolutionize the quality of their AI models.